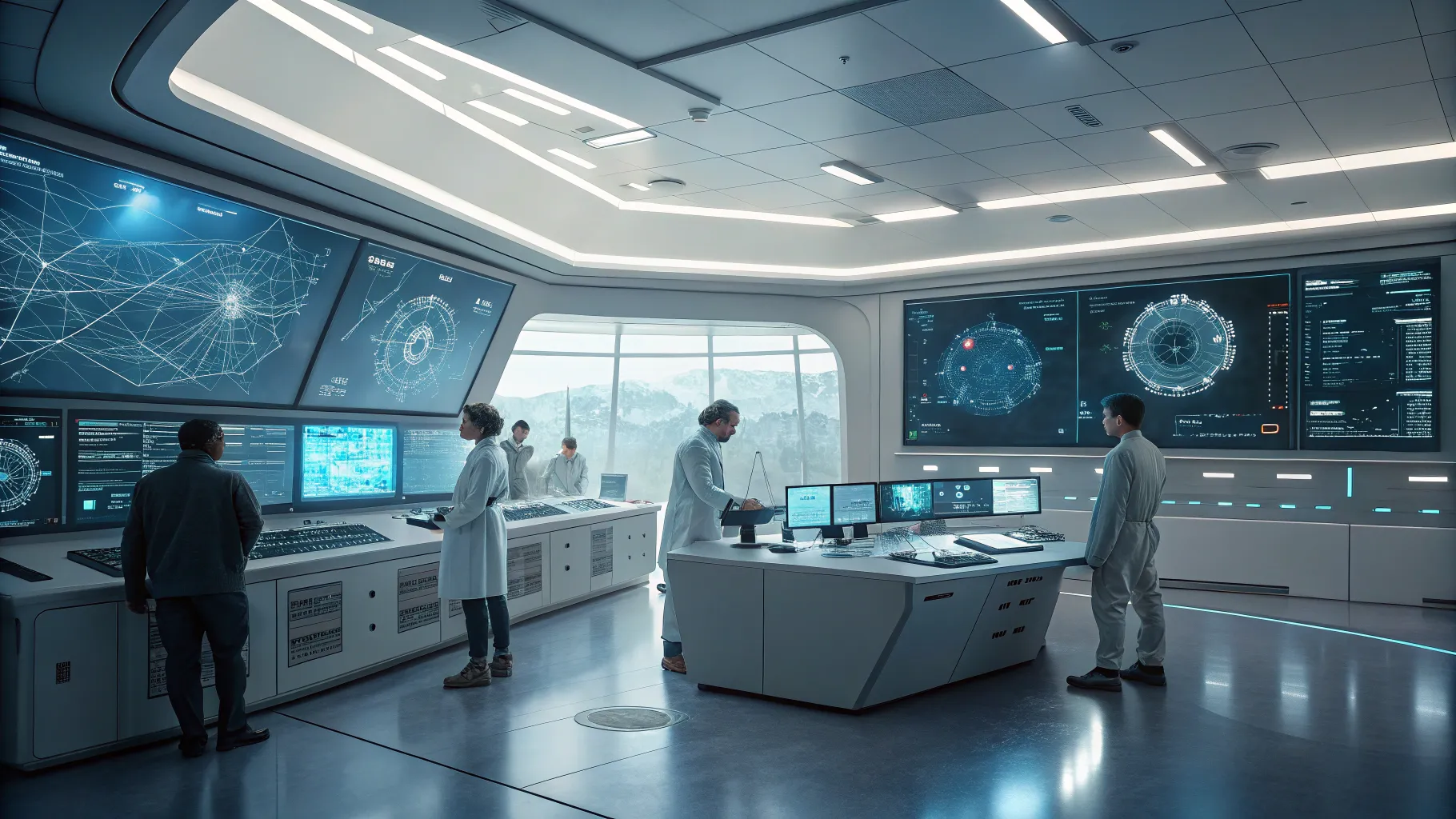
Recent analysis of artificial intelligence development strategies reveals that conventional wisdom about AI advancement may be flawed. Experts increasingly challenge the notion that all scaling approaches yield equal results in AI development, particularly questioning whether longer reasoning chains and increased computational power necessarily translate to higher intelligence.
The AI research community has long operated under certain assumptions about how to improve machine intelligence. However, new perspectives suggest these assumptions deserve closer examination as the field matures.
Rethinking Chain Reasoning in AI
One key finding challenges the belief that longer reasoning chains—where AI systems work through multiple logic steps to reach conclusions—automatically indicate more advanced intelligence. While step-by-step reasoning can help AI systems solve complex problems, the length of these chains does not necessarily correlate with the quality or sophistication of the AI’s thinking.
Some AI systems with shorter, more efficient reasoning patterns may actually demonstrate greater intelligence by quickly identifying optimal solutions rather than working through unnecessary steps. This suggests that the quality and efficiency of reasoning may be more important than the number of steps involved.
The Limits of Computational Power
Another significant insight questions the “more compute equals better AI” approach that has dominated the industry. While computational resources have been crucial to recent AI breakthroughs, experts now suggest that simply throwing more processing power at AI problems may yield diminishing returns.
The critique points to several limitations of the compute-centric approach:
- Increased energy consumption and environmental impact
- Limited accessibility for researchers with fewer resources
- Potential to mask fundamental algorithmic inefficiencies
Instead, researchers are beginning to focus on developing more efficient algorithms and training methods that can achieve comparable or superior results with less computational power.
Alternative Paths to Advanced AI
As the field reconsiders traditional scaling strategies, several alternative approaches are gaining attention:
Data quality over quantity: Rather than simply increasing dataset size, focusing on curating high-quality, diverse training data may lead to more capable systems.
Architectural innovations: Novel neural network designs might achieve better results than simply scaling up existing architectures.
Specialized training techniques: Transfer learning and few-shot learning could reduce the need for massive computational resources while improving AI capabilities.
These findings suggest that the AI research community may need to diversify its approaches to advancement rather than relying primarily on scaling existing methods.
The evolving understanding of AI scaling challenges researchers to think more critically about how we measure and develop machine intelligence. As the field matures, nuanced approaches considering multiple factors beyond size and computational power may prove more fruitful in creating knowledgeable systems.
This shift in thinking could have significant implications for the future direction of AI research. It could potentially make advanced AI development more accessible while reducing its environmental impact. It may also lead to systems that more closely mimic human intelligence, which often relies on efficient reasoning rather than brute-force computation.
Howie Jones
My name is Howie and I'm a Customer Success Manager at Calendar. I like to ensure our customers get the best experience using our product. If you have questions email me howie at calendar.com